Mistral AI just announced Codestral: a open-weight model designed for code generation tasks. It outperforms other models in a long-range eval for code generation and is fluent in 80+ programming languages. We test capabilities of the new Mistral's model on data analysis tasks, using the Code Interpreter SDK by E2B.
Codestral doesn't support using tools for code execution yet, so in this Python example, we added the code interpreting capabilities. We are going to build an AI agent that performs data analysis tasks on provided data, in a form of a csv file.
Why Code Interpreter SDK
E2B's code interpreter SDK quickly creates a secure cloud sandbox powered by Firecracker. Inside this sandbox is a running Jupyter server that the LLM can use.
In general, the Code Interpreter SDK allows you to build custom code interpreters. For example, you can install custom packages, have access to the internet, use the filesystem, or connect your cloud storage. The Code Interpreter SDK works with any LLM. (in this example, we are using it with the Mistral's newest model).
Key links
Overview
Installing dependencies
Defining API keys and prompt
Implementation of method for code interpreting
Adding tmethod for calling Codestral and parsing its response
Adding method for data upload
Putting everything together
Installing dependencies
We start by install the E2B code interpreter SDK and Mistral's Python SDK.
%pip install mistralai e2b_code_interpreter
Defining API keys and prompt
Let's define our variables with API keys for Mistral and E2B together with the model ID and prompt. We won't be defining any tools because Codestral doesn't support tool usage yet.
MISTRAL_API_KEY = ""
E2B_API_KEY = ""
MODEL_NAME = "codestral-latest"
SYSTEM_PROMPT = """You're a python data scientist that is analyzing daily temperature of major cities. You are given tasks to complete and you run python code to solve them.
Information about the the temperature dataset:
- It's in the `/home/user/city_temperature.csv` file
- The CSV file is using `,` as the delimiter
- It has following columns (examples included):
- `Region`: "North America", "Europe"
- `Country`: "Iceland"
- `State`: for example "Texas" but can also be null
- `City`: "Prague"
- `Month`: "June"
- `Day`: 1-31
- `Year`: 2002
- `AvgTemperature`: temperature in celsiu, for example 24
Generally, you follow these rules:
- ALWAYS FORMAT YOUR RESPONSE IN MARKDOWN
- ALWAYS RESPOND ONLY WITH CODE IN CODE BLOCK LIKE THIS:
```python
{code}
```
- the python code runs in jupyter notebook.
- every time you generate python, the code is executed in a separate cell. it's okay to multiple calls to `execute_python`.
- display visualizations using matplotlib or any other visualization library directly in the notebook. don't worry about saving the visualizations to a file.
- you have access to the internet and can make api requests.
- you also have access to the filesystem and can read/write files.
- you can install any pip package (if it exists) if you need to by running `!pip install {package}`. The usual packages for data analysis are already preinstalled though.
- you can run any python code you want, everything is running in a secure sandbox environment
"""
As an equivalent to missing function calling, we instruct the model to return messages in Markdown and then parse and extract the Python code block on our own.
import re
pattern = re.compile(r'```python\n(.*?)\n```', re.DOTALL)
def match_code_block(llm_response):
match = pattern.search(llm_response)
if match:
code = match.group(1)
print(code)
return code
return ""
Implementation of method for code interpreting
Here's the main function that use the E2B code interpreter SDK. We'll be calling this function a little bit further when we're parsing the Codestral's response with tool calls.
def code_interpret(e2b_code_interpreter, code):
print("Running code interpreter...")
exec = e2b_code_interpreter.notebook.exec_cell(
code,
on_stderr=lambda stderr: print("[Code Interpreter]", stderr),
on_stdout=lambda stdout: print("[Code Interpreter]", stdout),
)
if exec.error:
print("[Code Interpreter ERROR]", exec.error)
else:
return exec.results
Adding tmethod for calling Codestral and parsing its response
Now we're going to define and implement chat
method. In this method, we'll call the Codestral LLM, parse the output to extract any Python code block, and call our code_interpret
method we defined above.
from mistralai.client import MistralClient
client = MistralClient(api_key=MISTRAL_API_KEY)
def chat(e2b_code_interpreter, user_message):
print(f"\n{'='*50}\nUser message: {user_message}\n{'='*50}")
messages = [
{"role": "system", "content": SYSTEM_PROMPT},
{"role": "user", "content": user_message}
]
response = client.chat(
model=MODEL_NAME,
messages=messages,
)
response_message = response.choices[0].message
python_code = match_code_block(response_message.content)
if python_code != "":
code_interpreter_results = code_interpret(e2b_code_interpreter, python_code)
return code_interpreter_results
else:
print(f"Failed to match any Python code in model's response {response_message}")
return[]
Adding method for data upload
Now we implement a method to upload our dataset to the code interpreter sandbox. The file gets uploaded to the E2B sandbox where our code interpreter is running. We get the file's remote path in the remote_path
variable.
def upload_dataset(code_interpreter):
print("Uploading dataset to Code Interpreter sandbox...")
with open("./city_temperature.csv", "rb") as f:
remote_path = code_interpreter.upload_file(f)
print("Uploaded at", remote_path)
Putting everything together
In this last step, we put all the pieces together. We instantiate a new code interpreter instance using with CodeInterpreter(api_key=E2B_API_KEY) as code_interpreter:
and then call the chat
method with our user message and the code_interpreter
instance.
from e2b_code_interpreter import CodeInterpreter
with CodeInterpreter(api_key=E2B_API_KEY) as code_interpreter:
upload_dataset(code_interpreter)
code_results = chat(
code_interpreter,
"Plot average temperature over the years in Algeria"
)
if code_results:
first_result = code_results[0]
else:
raise Exception("No code interpreter results")
first_result
This is how the resulting plot generated by the LLM looks like, based on the dataset.
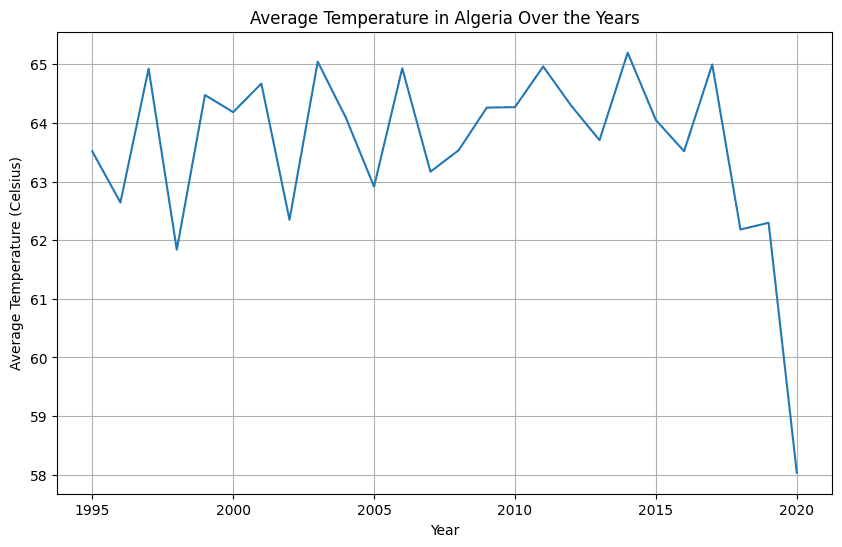
Key links